Someone is fidgeting in a long line at an airport security gate. Can data science tell us why? Is that person simply nervous about the wait?
Or is this a passenger who has something sinister to hide?
Even highly trained Transportation Security Administration (TSA) airport security officers still have a hard time telling whether someone is lying or telling the truth – despite the billions of dollars and years of study that have been devoted to the subject.
Now, University of Rochester researchers are using data science and an online crowdsourcing framework called ADDR (Automated Dyadic Data Recorder) to further our understanding of deception based on facial and verbal cues.
They also hope to minimize instances of racial and ethnic profiling that TSA critics contend occurs when passengers are pulled aside under the agency’s Screening of Passengers by Observation Techniques (SPOT) program.
“Basically, our system is like Skype on steroids,” says Tay Sen, a PhD student in the lab of Ehsan Hoque, an assistant professor of computer science. Sen collaborated closely with Kamrul Hasan, another PhD student in the group, on two papers in IEEE Automated Face and Gesture Recognition and the Proceedings of the ACM on Interactive, Mobile, Wearable and Ubiquitous Technologies. The papers describe the framework the lab has used to create the largest publicly available deception dataset so far – and why some smiles are more deceitful than others.
Game and data science reveal the truth behind a smile
Here’s how ADDR works: Two people sign up on Amazon Mechanical Turk, the crowdsourcing internet marketplace that matches people to tasks that computers are currently unable to do. A video assigns one person to be the describer and the other to be the interrogator.
The describer is then shown an image and is instructed to memorize as many of the details as possible. The computer instructs the describer to either lie or tell the truth about what they’ve just seen. The interrogator, who has not been privy to the instructions to the describer, then asks the describer a set of baseline questions not relevant to the image. This is done to capture individual behavioral differences which could be used to develop a “personalized model.” The routine questions include “what did you wear yesterday?” — to provoke a mental state relevant to retrieving a memory — and “what is 14 times 4?” — to provoke a mental state relevant to analytical memory.
Play the game: Can you tell which person is lying?
“A lot of times people tend to look a certain way or show some kind of facial expression when they’re remembering things,” Sen said. “And when they are given a computational question, they have another kind of facial expression.”
They are also questions that the witness would have no incentive to lie about and that provide a baseline of that individual’s “normal” responses when answering honestly.
And, of course, there are questions about the image itself, to which the witness gives either a truthful or dishonest response.
The entire exchange is recorded on a separate video for later analysis using data science.
1 million faces
An advantage of this crowdsourcing approach is that it allows researchers to tap into a far larger pool of research participants – and gather data far more quickly – than would occur if participants had to be brought into a lab, Hoque says. Not having a standardized and consistent dataset with reliable ground truth has been the major setback for deception research, he says. With the ADDR framework,the researchers gathered 1.3 million frames of facial expressions from 151 pairs of individuals playing the game, in a few weeks of effort. More data collection is underway in the lab.
Data science is enabling the researchers to quickly analyze all that data in novel ways. For example, they used automated facial feature analysis software to identify which action units were being used in a given frame, and to assign a numerical weight to each.
The researchers then used an unsupervised clustering technique — a machine learning method that can automatically find patterns without being assigned any predetermined labels or categories.
“It told us there were basically five kinds of smile-related ‘faces’ that people made when responding to questions,” Sen said. The one most frequently associated with lying was a high intensity version of the so-called Duchenne smile involving both cheek/eye and mouth muscles. This is consistent with the “Duping Delight” theory that “when you’re fooling someone, you tend to take delight in it,” Sen explained.
More puzzling was the discovery that honest witnesses would often contract their eyes, but not smile at all with their mouths. “When we went back and replayed the videos, we found that this often happened when people were trying to remember what was in an image,” Sen said. “This showed they were concentrating and trying to recall honestly.”
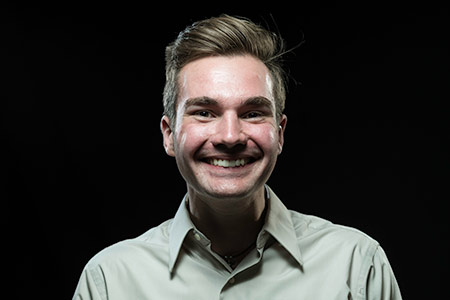
(University of Rochester photo / J. Adam Fenster)
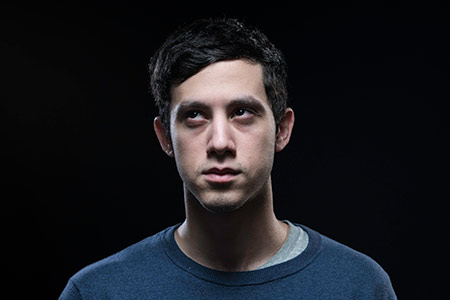
(University of Rochester photo / J. Adam Fenster)
Next steps
So will these data science findings tip off liars to simply change their facial expressions?
Not likely. The tell-tale strong Duchenne smile associated with lying involves “a cheek muscle you cannot control,” Hoque says. “It is involuntary.”
The data science researchers say they’ve only scratched the surface of potential findings from the data they’re collected.
Hoque, for example, is intrigued by the fact the interrogators unknowingly leak unique information when they are being lied to. For example, interrogators demonstrate more polite smiles when they are being lied to. In addition, an interrogator is more likely to return a smile by a lying witness than a truth-teller. While more research needs to be done, it is clear that looking at the interrogators’ data reveals useful information and could have implications for how TSA officers are trained.
“There are also possibilities of using language to further decipher the ambiguity within microexpressions.” Hasan says. Hasan is currently exploring this space.
“In the end, we still want humans to make the final decision,” Hoque says. “But as they are interrogating, it is important to provide them with some objective metrics that they could use to further inform their decisions.”
Kurtis Haut, Zachary Teicher, Minh Tran, Matthew Levin, and Yiming Yang – all students in the Hoque lab – also contributed to the research.