Early results show 98 percent accuracy in predicting malignant tissue.
Mammography is the gold standard for breast cancer diagnosis, but it’s not reliably accurate in all cases, especially in people with dense breasts. At the University of Rochester, Avice O’Connell ’77M (Res) a professor of imaging sciences at the Medical Center, and Kevin Parker, a professor of electrical and computer engineering at the Hajim School of Engineering & Applied Sciences, wanted to do better. Along with Jihye Baek, a PhD student in electrical and computer engineering, they launched a research project incorporating ultrasound with machine learning for previously detected masses. The end result: nearly 98 percent accuracy in predicting breast cancer in these masses.
The sensitivity of mammography can range from 40 percent for dense breast tissue to a high of just above 90 percent. “While 90 percent sounds good, a higher accuracy rate means we can help many more women,” says Parker.
The findings were published last November in the journal Machine Learning: Science and Technology. Parker’s team combined ultrasound with a feature-based machine-learning method for breast cancer detection, adding a color overlay map showing the probability of malignancy.
Mammography versus breast ultrasound
Mammography and ultrasound are the two main methods of breast cancer diagnosis. Mammography relies on an x-ray system to take an image of breast tissue. Breast ultrasound, as the name suggests, produces an image of breast tissue—a sonogram—from sound waves. A handheld wand called a transducer scans the breast with sound waves, and the resulting sound echoes create the image.
As the researchers point out in their paper, ultrasound is less expensive than mammography, portable, and radiation-free, making it a desirable tool, especially in developing countries. But at present, ultrasound is used only as a complement to standard mammography. That’s because, as O’Connell explains, ultrasound finds many masses within a breast, most of which are not cancer. “Breast imagers need new ways to reduce the number of false positive biopsies,” she says.
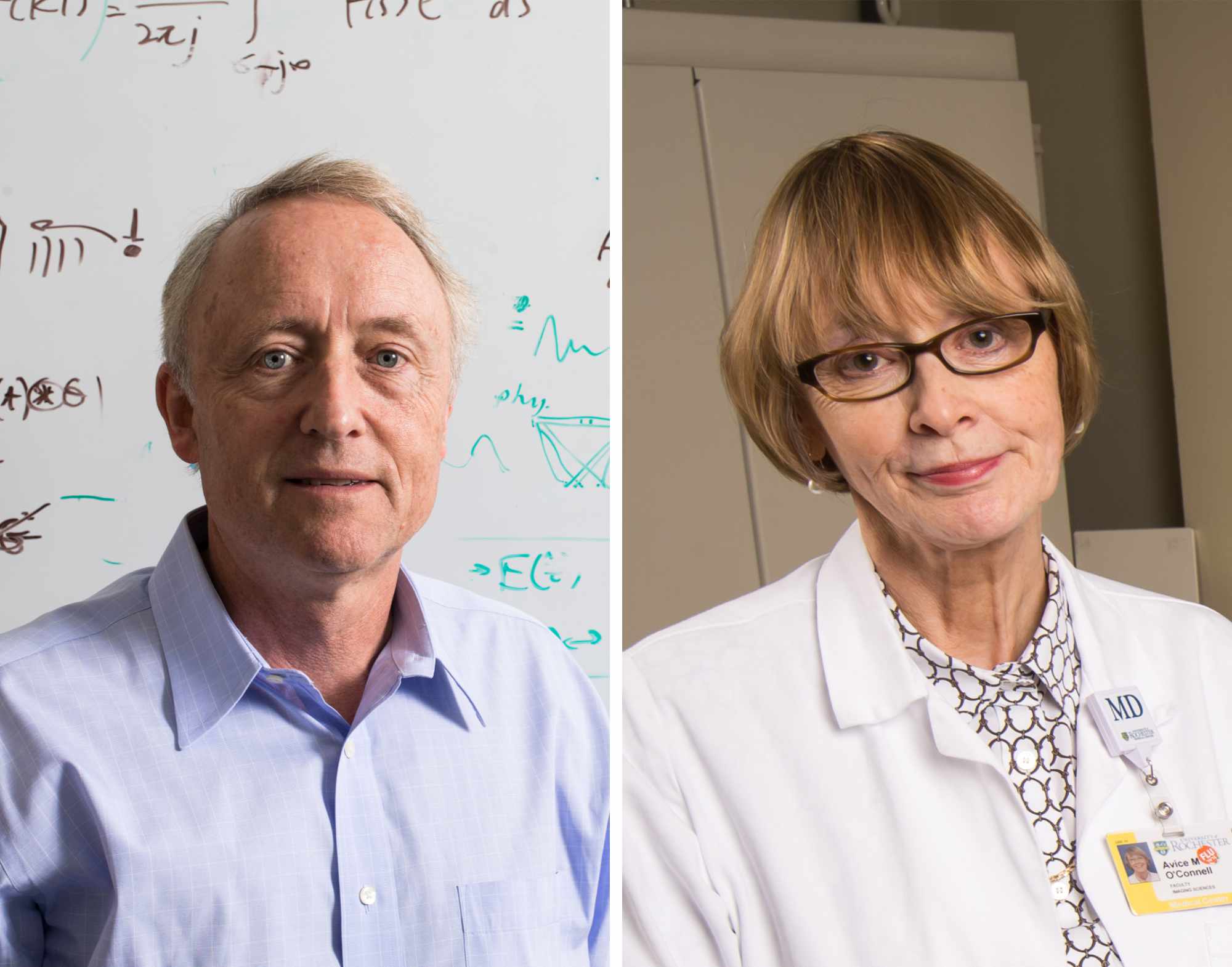
Ultrasound better than mammography at imaging dense breast tissue
Improving breast cancer screening and diagnosis is an active area of research locally and nationally. At the Medical Center and its Wilmot Cancer Institute, for example, a unique 3D ultrasound system has been developed separately for patient care and continues to be evaluated.
Improved ultrasound diagnostics are especially important for women with dense fibroglandular tissue. According to the National Cancer Institute, that includes nearly half of all women age 40 and older who get mammograms. “For them, the sensitivity is as low as 50 percent with mammograms,” says Parker. “With advanced ultrasound, we may be able to get into the upper 90 percentiles of accuracy.”
Parker believes their system also has unique features in that it simplifies the process. While some researchers are looking at many features in the breast—some as many as 30—the Rochester team focuses on five key biophysical features involving the distribution of cells, blood vessels, and proteins.
Machine-learning ultrasound: a promising new means to improve diagnosis, reduce false positives
The team’s approach involves machine learning, which is a type of artificial intelligence, or AI. From the data the researchers input, the computers develop algorithms that enable them to learn patterns and better recognize cancerous tissue. The new image that is produced by the algorithm shows the probability of malignancy within lesions, using a color overlay with blue and green corresponding to benign tissue and red corresponding to a high probably of malignancy.
Parker and O’Connell are pleased with the 98-percent accuracy rate, but they note it was accomplished with only 121 scans of suspicious breast lesions from patients at the University of Rochester Medical Center.
“In the next stage of our research, we’ll be working with much larger sets of data,” says Parker.
Given the need for more data, it’s difficult to say how quickly their framework for computer-assisted ultrasound can be put into practice. O’Connell says, “More research is needed on many more patients for this to be widely adopted.”
But that’s how any task based on machine learning works. Computers get “smarter” with more data, producing more precise algorithms, translating into better diagnostics, and in the case of breast cancer screening, more lives saved.
Improving diagnostics in developing countries
Because ultrasound is portable and less expensive than mammography, it’s a desirable tool in developing countries. But it still requires an experienced sonographer.
In a separate research paper published in PLOS Digital Health, by Tom Marini ’17M (MD), a clinical instructor in the Medical Center’s Department of Imaging Sciences, along with Parker, O’Connell and their collaborators, the authors describe the possibility of combining a type of ultrasound—called volume sweep imaging (VSI)—with artificial intelligence to provide an automatic and inexpensive form of breast mass diagnosis. Since VSI does not require an experienced sonographer, they hope this approach can make a difference in low- and middle-income countries, where access to breast cancer diagnosis and care poses a challenge.
Read more
New imaging technology could buy time for pancreatic cancer patients
Tumor shrinkage is one sign of cancer treatment’s efficacy—but Rochester scientists are exploring elasticity and permeability as well.
Breakthrough adds new color to ultrasound.
Rochester engineering professor Kevin Parker has devised a way to differentiate fine details in medical ultrasound images that currently appear as indistinguishable objects in shades of gray.
Novel imaging system could mean near-instant biopsy results
The new imaging system developed by Michael Giacomelli, an assistant professor of biomedical engineering and of optics, uses two-photon fluorescence microscopy (TPFM).