The combination of drinking, social media, and sharing has provided Rochester researchers with an innovative test case for analyzing ongoing behavior by Twitter users and then using this analysis to study patterns about drinking in different communities.
In a new paper, PhD student Nabil Hossain reports that he and his collaborators have taught computers to analyze tweets about drinking in an effort to predict where Twitter users are when they report drinking.
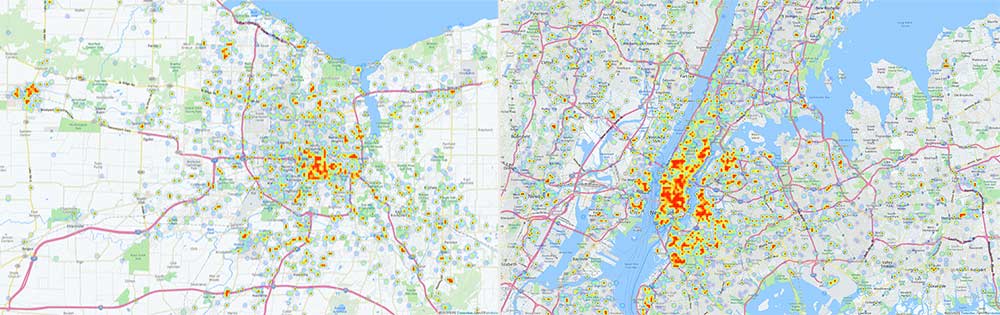
Hossain is a student in the computer science group led by Henry Kautz, the Robin and Tim Wentworth Director of the Goergen Institute for Data Science. He posted the paper on the arXiv.org repository after it was accepted for the International AAAI Conference on Web and Social Media to be held in Germany in May. An article in MIT Technology Review, which has helped Hossain’s work find a viral life of its own, says the work is based on “two breakthroughs.”
“The first is a way to train a machine-learning algorithm to spot tweets that relate to alcohol and those sent by people drinking alcohol at the time,” according to the article. “The second is a way to find a Twitter user’s home location with much greater accuracy than has ever been possible and therefore to determine whether they are drinking at home or not.”
For the first step, the researchers chose to use alcohol consumption to demonstrate the effectiveness of their model, which can not only distinguish between people who are discussing an activity versus those who are discussing performing the activity themselves, but can also determine whether they are performing it at-the-moment as opposed to some time in the past or the future. This model could also be applied to other behaviors, not just drinking.
Until now, predicting a social media user’s home location was done by establishing the place from which the user most frequently tweets, or the most common last location of the day from which the user posts. In the new work, the researchers applied machine-learning techniques to identify in-the-moment user behavior. That allowed them to accurately predict users’ home locations within 100 meters.
Combining these tools, they were able to discover patterns of alcohol use in urban and suburban settings. Their goal is that “such methods can help us better understand the occurrence, frequency, and settings of alcohol consumption, a health-risk behavior, and can lead to actionable information in prevention and public health.”