A data science project to help clinicians predict Parkinson’s disease progression landed two University of Rochester undergraduates and their faculty mentor a top spot in a prestigious data science contest.
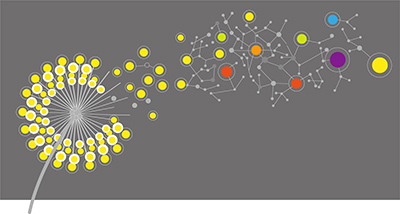
Unlocking big data
A Newscenter series on how Rochester is using data science to change how we research, how we learn, and how we understand our world.
Sam Lerman ’17, a dual computer science and mathematics major, and Nick Potter ’17, a mathematics major, led the project in collaboration with Charles Venuto, an assistant professor of neurology at the Medical Center’s Center for Human Experimental Therapeutics. The team entered their computer model in a data challenge organized by the Michael J. Fox Foundation and GE Healthcare. Competing against other data and computational scientists, the students learned their submission was one of the top three finalists in determining baseline factors predictive of clinical disease progression in Parkinson’s disease patients.
“This is quite the impressive accomplishment for our students and research,” Venuto says. “Identifying disease progression in Parkinson’s disease has been a real challenge. Right now, clinicians lack objective means to provide Parkinson’s disease patients advice on what to expect the symptomatic course of their disease will be in the next six months, in the next year, and so on.”
Parkinson’s disease is a nervous system disorder that affects movement, with symptoms worsening over time. Age of onset, rate of disease progression, and type and severity of symptoms vary for the five million people worldwide living with the disease.
Generating algorithms and models for prognosis would aid in patient care and planning clinical trials.
The Parkinson’s Progression Markers Initiative (PPMI) provided contest participants with a dataset of baseline information from a group of patients. The challenge involved analyzing this dataset to gain new insight into diagnosis and disease progression.
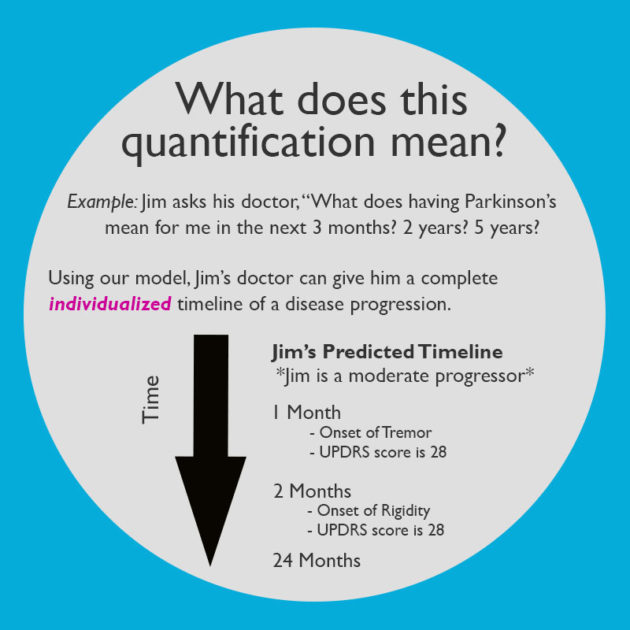
“First we asked, ‘How is disease progression quantified?’” Lerman says. “Once we defined our target, then we worked on developing and building models.”
Lerman, Potter, and Venuto’s submission included machine learning algorithms they developed to predict three things:
- Rate of progression: Will patients have fast, moderate, or slow disease progression?
- Time until symptom onset: How long, in months, will it take for a patient to exhibit tremors or bradykinesia—a symptom characterized by slowness of movement?
- Future score on disease scales: How will a patient fare on the Unified Parkinson’s Disease Rating Scale (UPDRS), a rating tool to measure disease progression through analysis of mood, behavior, activities of daily living, and motor skills?
“The machine learning side of it was programmed abstractly so that this can be applied to any diseases that have these similar clinical outcome scale measurements,” Lerman says. These could include Huntington’s and other neurodegenerative diseases.
In the future, they envision a prognostic tool and interface that a clinician could use when interacting with patients.
“Our hope for the next step is that when a patient goes to his or her doctor and asks, ‘What can I expect from this disease?’ the doctor can easily run the program to create an estimated timeline of the patient’s disease,” Lerman says.