University team leads competition for best computer-generated captions
A team of University and Adobe researchers is outperforming other approaches to creating computer-generated image captions in an international competition. The key to their winning approach? Thinking about words – what they mean and how they fit in a sentence structure – just as much as thinking about the image itself. The Rochester/Adobe model mixes the two approaches that are often used in image captioning: the “top-down” approach, which starts from the “gist” of the image and then converts it into words, and the “bottom-up” approach, which first assigns words to different aspects of the image and then combines them together to form a sentence. The Rochester/Adobe model is currently beating Google, Microsoft, Baidu/UCLA, Stanford University, University of California Berkeley, University of Toronto/Montreal, and others to top the leaderboard in an image captioning competition run by Microsoft, called the Microsoft COCO Image Captioning Challenge. While the winner of the year-long competition is still to be determined, the Rochester “Attention” system – or ATT on the leaderboard – has been leading the field since last November. Other groups have also tried to combine these two methods by having a feedback mechanism that allows a system to improve on what just one of the approaches would be able to do. However, several systems that tried to blend these two approaches focused on “visual attention,” which tries to take into account which parts of an image are visually more important to describe the image better. The Rochester/Adobe system focuses on what the researchers describe as “semantic attention.” In a paper accepted by the 2016 IEEE Conference on Computer Vision and Pattern Recognition (CVPR), entitled “Image Captioning with Semantic Attention,” computer science professor Jiebo Luo and his colleagues define semantic attention as “the ability to provide a detailed, coherent description of semantically important objects that are needed exactly when they are needed.”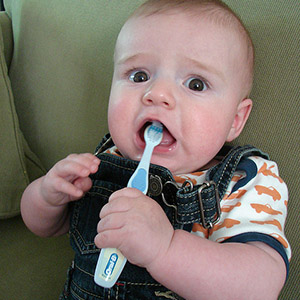
Rochester ATT caption: “A baby with a toothbrush in its mouth.”
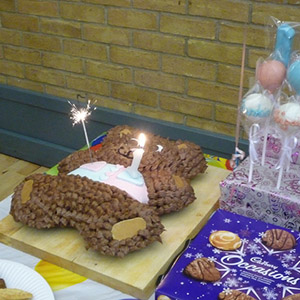
Rochester ATT caption: “A table topped with a cake with candles on it.”
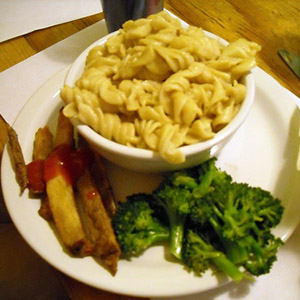
Rochester ATT caption: “A plate with a sandwich and french fries.”