The students analyzed millions of data points to determine where to best place fire stations, fire trucks, and other resources.
Where should the Rochester Fire Department (RFD) locate fire stations over the next 10 years to minimize response times to emergencies? Where should it deploy the most fire trucks? How do seasonal shifts impact the nature and volume of emergencies that firefighters need to respond to?
These are questions that a team of students from the University of Rochester’s Goergen Institute for Data Science and Artificial Intelligence explored over the course of a semester for their capstone project sponsored by the RFD.
“We were looking for someone from the outside to come in and provide a different set of ideas, experiences, and viewpoints to help us enhance our emergency response services,” says Daniel Curran, a captain for planning and research who is responsible for technology-based projects at the RFD. “We felt the students would have an unbiased perspective and look at the situation and tell us, ‘This is where the data leads us,’ and come to their own conclusions.”
Over the course of the semester, the students used artificial intelligence and other data analytics tools to make sense of more than 1.6 million points of data collected by RFD from 2006 to 2024. They also incorporated external census data related to population, income, property, and housing to enrich their analysis.
“This was an amazing opportunity to contribute something meaningful,” says data science graduate student Brynn (Ye In) Lee ’24 (MS). “The RFD has 15 fire stations, they’re supporting about 500 personnel, and they have around 50,000 annual dispatches, so that’s a huge impact that we can have on the community.”
The students developed interactive maps that allow the RFD to analyze how long it takes the firefighters to reach an incident, while providing information about the distribution of incidents across the city and the ability to sort by incident type. They also created models to predict the monthly incident density over the next 10 years for all 15 fire stations.
In their analysis, the students found that the RFD is already effective at responding to incidents in a timely fashion. However, the students offered recommendations for small improvements that could further decrease response times. Their suggestions include reallocating specific types of trucks from one station to another and introducing programs similar to those in other cities that can address non-life-threatening calls with fewer resources.
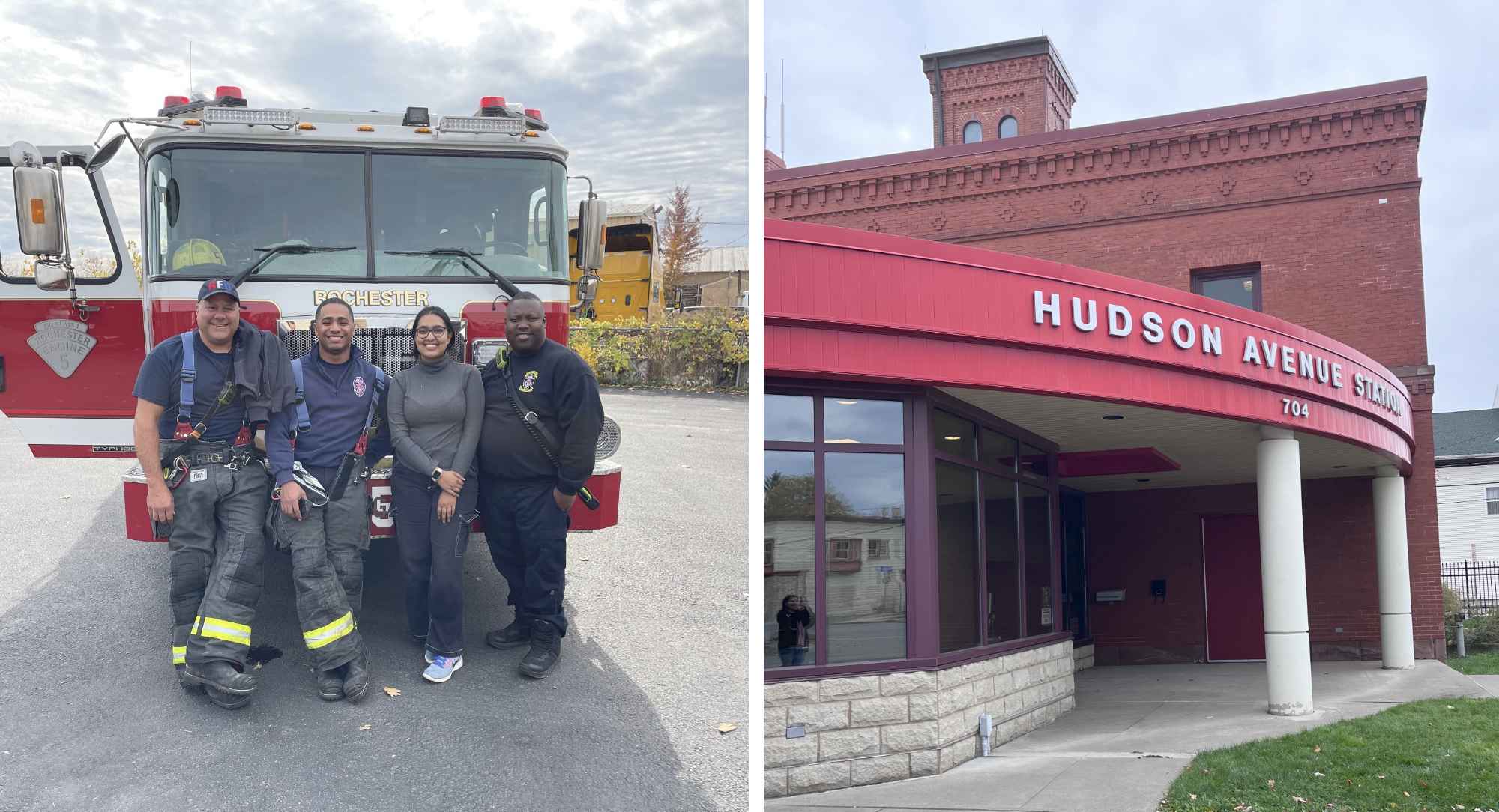
According to the students, a critical aspect to their project’s success was going on “ride-alongs” with the RFD, which gave them an appreciation for the firefighters’ day-to-day responsibilities. They said witnessing the types of incidents the firefighters respond to, learning about their shifts, and seeing the equipment in person was enlightening.
“I used to think the fire department only deals with fire-related emergencies, but they deal with so many medical emergencies and spend a lot of time early in the morning patrolling their area to look for fire hazards,” says Homayra Tabassum ’24 (MS). “Getting to see that in person helped us be much more insightful when we were thinking about resource allocation.”
The students said their weekly meetings with the RFD sponsors and collaborating with RFD’s internal data analysts were important learning opportunities as well.
“Dealing with real-world data is not always clean or exactly the way you want it, so the captain and the senior data analysts were extremely helpful,” says team member Medhini Sridharr ’24 (MS). “They helped us choose the most important variables based on their domain knowledge, which was crucial because we had more than 300 variables to consider. They helped us drill down to what’s important.”
Overall, the students said they loved the chance to do a hands-on project with real-world implications, witness the firefighters at work first-hand, and deliver a product that will serve the RFD and local community for years to come.
The project team included data science master’s students Eugene Ayonga ’24, Lee, Sridharr, Tabassum, as well as undergraduate data science student Nour Assili ’26.